Retailers are increasingly embracing AI-based solutions for full lifecycle pricing, that provide price, promotion and markdown optimization that keeps pace with rapidly changing competitor, market and shopper behaviours that cannot be predicted by just looking at the past.
But there are important considerations that are often overlooked in selecting a state-of-the-art pricing tool and ensuring successful adoption that is crucial to achieving business and financial goals.
Successful retailers will take a comprehensive approach to prepare their organization to be data-driven, including:
1. Plan for the initial data hygiene and ongoing data maintenance that will continue to unlock value from the optimization solution.
I think of price optimization as being a lot like buying a new car – when you first drive it, it’s incredibly exciting, and you see a big difference between its performance and that of whatever legacy machine it replaced. But if you haven’t invested in starting with clean, comprehensive data and in keeping that data current and accurate going forward, you see diminishing returns. Over weeks, months and years of failing to maintain the data, you fall farther and farther behind more disciplined competitors and lose touch with what excited you in the first place, namely delivering prices and promotions that meaningfully engage your shoppers.
The pricing teams need to be trained so they have the skills to set guardrails that capture meaningful anomalies, like if a recommended price went up by more than X% because cost rose sharply – the equivalent of a ‘check engine’ light that captures your attention. Did someone perhaps enter a mistaken cost? Or did that item really experience an abrupt cost increase?
But if users over-constrain the system with too many rules and too-narrow constraints, they are keeping the data science from doing its job effectively. As they gain experience with the AI-based solution, they should reduce the number of business rules and constraints to let the science deliver more and more pricing autonomy as it learns in that retailer’s real-world conditions. In a modern automobile, engines are specifically tuned to optimal performance in several areas. Any further constraints make the performance less optimal. In my experience just 10-15 rules per category provides optimal results.
When the pricing team is well-trained, supported by the IT team to have good data maintenance and flows, and continue to move up the maturity curve in leveraging data science, the category managers have the confidence that they are driving a well-maintained, reliable vehicle that won’t break down and that will last. The reward is continuing ROI to the overall organization as categories achieve their revenue, margin, and profit goals more consistently.
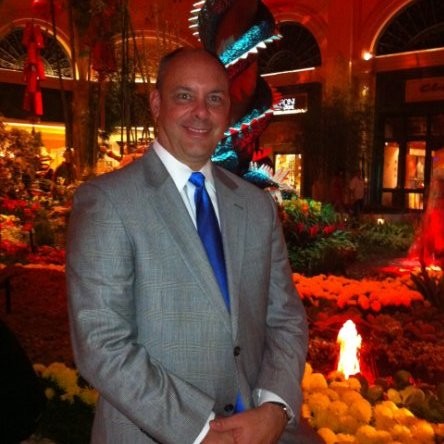
2. Take advantage of the system’s insights on cross-item effects.
Seasoned price strategists and category managers have strong beliefs about which items have affinity, where promoting an item and taking a margin hit will drive sales of other higher-margin items to more than offset the reduced price, and which cannibalize, where promoting one item may boost units and revenues for that item but will undercut sales of other substitutable items that may do more harm than good to the category overall. Over many years of helping organizations harness and effectively adopt the data science in optimization tools, I’ve seen the disconnects between long-held beliefs about affinity and cannibalization v. the reality that AI-based tools reveal.
This is particularly true when it comes to Key Value Items (KVIs), which are the items where shoppers pay most attention to price and which have a significant impact on price image. For example, when a category manager and price strategist see a system-recommended price reduction on a KVI they believe strongly in, say a popular brand of breakfast cereal, they may be resistant to lowering a price on what is a lucrative item in the category. But the science factors in elasticity and the current, accurate demand signals, and adopting the recommendation may in fact make the retailer more of a destination, driving overall basket size and foot traffic and improving their price image. In other words, lowering a price on one item may drive more revenue and margin for the retailer overall. Seeing the science in action and the measurable results it delivers starts to build the trust, category by category, and increases overall adoption and ROI.
3. Embrace the power of simulations and what-if scenario planning.
One of the most powerful ways to gain the benefits of AI-based data-driven pricing is to leverage simulations and what-if scenario planning. Think of these as test drives, and you are evaluating the pros and cons of each. The pricing and category management teams can collaborate to see how changing an item strategy would impact margins, units and profits, for example. Similarly, price strategists can explore how to increase traffic by increasing the importance of unit sales in the model while being more willing to sacrifice margins, and the data science will tell them what price would support that goal. These capabilities provide the ability to grow more fluent with the tool, more confident in the science, and more innovative in the market to most effectively engage shoppers while meeting overall financial and business goals.
Taken together, these proven strategies enable retailers to more rapidly and effectively leverage the potential of a leading price, promotion and markdown optimization suite. With some planning and discipline around data, and an open and curious approach to cross-item effects and simulations, pricing teams and category managers can deliver transformative – and measurable – positive impact for their businesses.