Artificial Intelligence (AI) dominates today’s news cycle, and for good reason. It is both a catalyst of major change in numerous industries, and a technology upon which significant negative sentiment is growing around its future applications.
But we must be careful not to consider ‘artificial intelligence’ as a blanket term, because it simply isn’t. Putting the specifics of their programming aside, it’s safe to break today’s AI tools into two key types, each with its own focus and methodology.
The problem with generative AI
Headline-grabbing applications like ChatGPT and DALL-E are made possible through what’s called generative AI. These make use of large language models (LLMs), which utilise a deep catalogue of data drawn from all of history, including sources like books, encyclopaedias and the general web. These can then be prompted with natural language to create new text-based content, realistic-looking synthetic data, or digital images like generative art.
The controversy surrounding such systems stems from their ability to create potentially undetectable forgeries like deepfake images and video, documents which look authentic but may contain misleading information – or, worse, the perceived potential of AI-powered chatbots to generate and spread disinformation. Technologically rapid development in a context of a lack of industry guidelines and government regulation has sown the seeds of deep-rooted suspicion.
Used correctly, though, generative AI has numerous practical business applications in industry, commerce, education, and healthcare provision. As a customer service front-end, generative AI is ideal as it already knows the answers given the appropriate data set. Major technology companies are gradually increasing its presence in their services, and AI is helping today’s businesses weather tough economic times by taking over certain key functions. Some analysts even go as far as calling AI the beginning of a new Industrial Revolution. But today’s generative AI also has significant limitations.
Firstly, generative AI must be trained on a huge data set, the size and scope of which limits its knowledge. Its output can be very accurate or (in a very simplified sense) highly educated guesswork, but it needs constant updating or its output will be out of date or just plain wrong. Secondly, generative AI produces content which looks or reads like the real thing, but its algorithmic engine is concerned only with putting words or pixels in the correct order – today, it doesn’t know the factual accuracy of the content it generates.
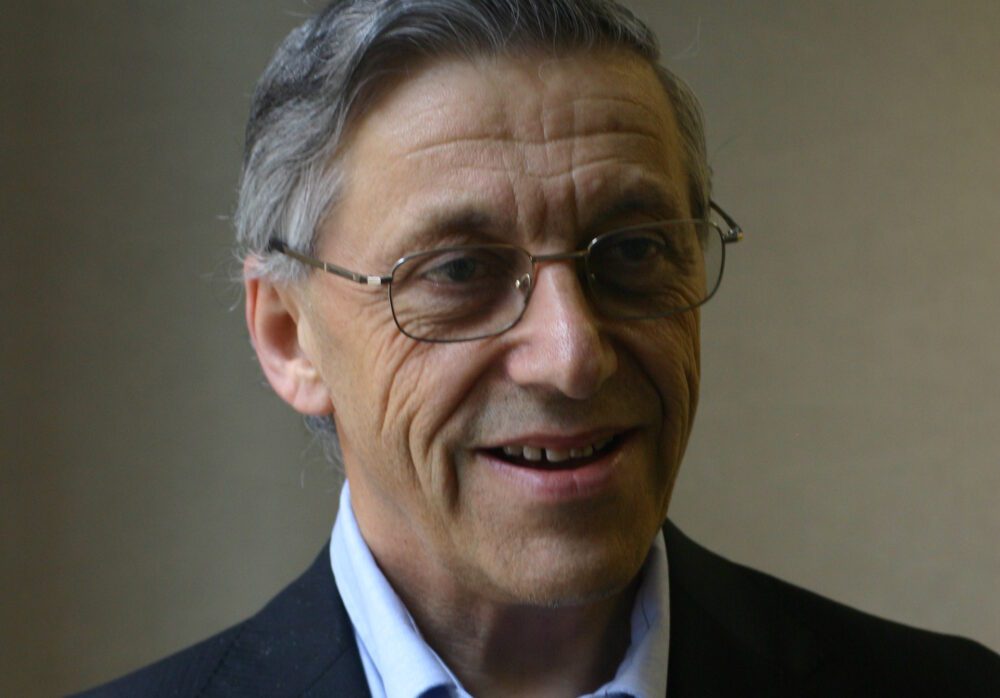
The power of operational AI
When business data is the focus, as it tends to be when looking to optimise retail processes, we turn to what we’ll call operational AI. This kind of model does not generate new or original works. Instead, operational AI is focused on analysis and classification of the data it is given. It deals in absolutes, applying its algorithms to live, true figures, and outputting valuable real-world data in return – the kind of data which can enhance the efficiency and performance of retail activities. AI can help automate processes, simulate marketing strategies, and optimise pricing and promotions.
Generative AI, for all its positives, cannot easily be applied in the same way, at least not yet. For example, it could be asked to generate dummy data for planning or training for a specific business, but this would have little basis in reality – whereas operational AI can perform detailed simulations based on past trends, current figures, and future events, creating meaningful predictive data with which to plan for smooth and profitable retail operations.
Better retail business through AI tools
Operational AI does not dominate the headlines in the same way as engines like ChatGPT, but countless organisations have already deployed it to forecast demand, optimise prices and benefit from predictive sales insights. Indeed, AI’s use in business analytics has existed for the best part of a decade. Over that time, much has been learned, which means its power is increasing all the time. Despite its age, operational AI is in its relative infancy, and similar to generative AI, we are only seeing a fraction of its potential today.
Increasing processing power and advances in AI science and technology have led us to the point at which existing AI models can be re-platformed and powered up, creating the opportunity to run many different algorithms concurrently. The data we have gathered about the way such models work leads to new, advanced models. These are even more adept at automatically predicting trends, aiding in sales planning, providing immediate shop-floor feedback, and optimising prices to meet customer desires – all while pre-set guard rails and parameters ensure the accuracy of the AI-powered system’s recommendations.
Next-generation AI technology
In the next few years, the potential of retail AI will grow further still. Planning, negotiations and pricing work, which today might require many staff and countless hours to administer, could be automated and optimised by these smarter, more reliable, more accurate AI tools (an ensembled use of several different AI algorithms) that require only minimal oversight.
Trusted AI models will be able to handle negotiations with suppliers, price the product and drastically reduce the complexity of large retail operations while leaving staff to focus on more strategic work, as well as saving head count through greater productivity. Walmart is trialling a pilot scheme whereby an AI bot engages in negotiations for the purchase of products from non-resale suppliers – something that three out of four suppliers say they prefer.
AI can improve efficiency significantly. The models can incorporate customer, pricing, promotions, loyalty, advertising, stock and assortment data – and the models then support algorithms that provide the kind of insights that ensure exceptional retail performance through the optimisation of prices, promotions and the go-to-market strategy. The impact will be felt from the head office to the shop floor, and from the distribution centre to the store stock room.
Already, forward-thinking companies are using AI to manage many of these functions to ensure they have the right products in the store, at the right price, with the supply chain set up to ensure optimal shelf stock levels, optimise delivery and unloading to ensure gaps are filled as swiftly as possible.
While today’s AI world is one of research and development, improvement, and increasing power, the far future may change things completely. The concept of artificial general intelligence (AGI) combines numerous AI models of different kinds to create what is essentially a representation of human intelligence in software. An AGI is not limited by its programming or the narrow scope of a single task. It can adapt and automatically find solutions to unfamiliar tasks. But while AGI may be, according to experts, years, decades, or centuries away, operational AI, conversely, has the power to revolutionise retail today.
Generative AI’s negative headlines may be off-putting, but it is clearly only one side of the AI coin. Embracing the power of operational AI is the way to discover incredible and immediate profitability gains, find new efficiencies through automation, and master marketing strategy. Given the pace of development, the potential of tomorrow’s retail AI models will be even greater.
Discover more: https://www.retailexpress.com/solutions/end-to-end-merchandising/
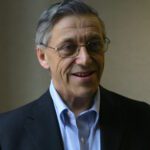
Barry Grange
Barry began his retail career with Tesco where he held several appointments including Systems Director, Management Services Director and Group CIO. Moving to the US, Barry became Executive Vice President of a leading worldwide supply chain provider, following which he established British American Consulting Group, operating as Chairman and CEO for 10 years. The business was sold to venture capitalists due to its major shares in the Retail ERP market, with over 50 leading retailers worldwide. Barry established Retail Express to address the shortcomings within retail merchandising, creating RE 2.0 to bring together years of retail knowledge and experience, a scientific approach to retail problem solving, best practice and technology.