Businesses that fail to deliver personalized recommendations risk losing customers to competitors who do. These recommendations can enhance the customer experience and improve sales. According to a report, businesses that offer customized experiences see an average 20% increase in sales. On the other hand, poor recommendations may result in customer dissatisfaction, reduced engagement, and, finally, losses.
A content recommendation engine is an ideal solution to offer these experiences. It can analyze customer behavior and previous purchases and recommend ‘related items.’ However, the customers’ expectations for recommendations are even higher.
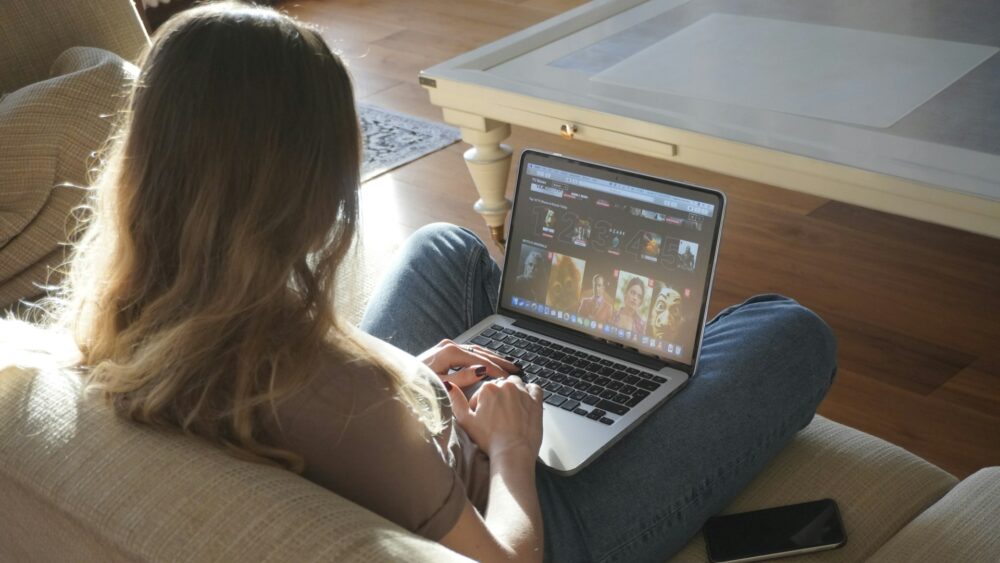
Businesses can capitalize on content recommendation engines integrated with advanced technologies to adapt to this change in customer behavior.
Here’s how advanced technologies like AI/ML enable content recommendation engines to deliver highly personalized content.
How Do Content Recommendation Engines Work?
Content recommendation engines use customer information to recommend content users want. This data includes:
- Implicit Data: These are the data based on customer engagement, such as search history, clicks, and order history.
- Explicit Data: The demographics and psychographics of the customer.
- Product Catalog Data: Information related to a product, such as descriptions, categories, genres, tags, and prices.
The gathered data is filtered using content-based and collaborative techniques to find similarities in a customer’s preferences. Content recommendation engines use this data to ensure users receive the most relevant content.
The engine remains active to ensure the delivery of appropriate content recommendations at the right time. This continuous learning provides more data to the engine, and the recommendations will get more accurate over time. It also incorporates positive and negative feedback from users to refine the recommendation process.
However, if the engine does not have real-time interaction capabilities, it may produce irrelevant suggestions and cause customer inconvenience. It may also ignore factors such as sensory appeal and broader product context in the recommendation process.
An AI-powered recommendation engine can address and mitigate these drawbacks. It can analyze patterns and adapt to changes instantly to improve suggestions.
Role of AI/ML in Enhancing Content Recommendation Engines
A content recommendation engine powered by artificial intelligence goes a step further and provides personalized suggestions. AI/ML enables businesses to understand their customer’s preferences with a continuous learning process.
This feature is crucial for companies wanting to stay relevant and deliver meaningful recommendations.
Here’s how AI/ML is transforming the traditional content recommendation engine into an advanced AI recommendation engine and providing hyper-personalized experiences.
How AI/ML Can Adapt to Critical Scenarios
AI helps recommendation engines solve various challenging scenarios. This includes high-frequency catalog updates and new product introductions with little historical information. AI-powered NLP enables engines to deliver highly relevant product recommendations. It focuses on less frequently browsed or purchased items, such as low-traffic or seasonal products. NLP extracts attributes from the product description (even reviews) and finds related products based on feature complements, semantic similarity, and word vectors.
Further, image-based recommendations can use AI to recommend products similar to the customer-provided image. It can also highlight the products that complement the image even if the customers have never interacted.
Optimizing and Automating Merchandising
AI-powered recommendation engines can automate and optimize suggestions for cross-selling, upselling, and ‘completing the bundle.’ Sophisticated data processing analyzes product portfolios to ensure compatibility with current stock. It automates the delivery of more targeted product offerings to customers to increase sales conversion rates.
Artificial intelligence enables businesses to define their merchandising rules with ease. They can easily expand their product range as AI handles item compatibility, eliminating the need for manual matching. It also allows the engine to factor dynamically in the product availability to provide recommendations.
Real-Time Personalization Across Channels
Personalization isn’t exclusive to a single channel; it must span all touchpoints. The modern recommendation engines use on- and offline data to provide an real-time, unified user profile. It is the basis for a highly targeted approach to customer interactions with a company and the brand across the web, app, and physical stores.
Regardless of where the consumer interacts with the brand, the recommendations change in real-time based on the user’s activity. The engine ensures the consumer receives relevant and coherent content in every channel.
Controlled Decisions in AI Recommendation Engines
One of the significant challenges for businesses is understanding the impact of AI-driven decisions. Modern recommendation engines give visibility into how suggestions are generated and how they affect the selected key performance indicators. These engines also explain why a particular algorithm is used and how it is performing. This will help the business understand its personalization strategies better and make necessary changes.
Companies can use this to refine the recommendation engine based on several KPIs, such as conversion rates or customer engagement, all the while having confidence in the ability of the algorithm to make the right decisions.
The Future of Content Recommendation Engines
With the focus on individual consumers and more attention paid to customer experience, the value of AI/ML applications has only grown. It can give precise insight into customer behavior and equip recommendation engines for engagement and conversion. These advancements can also achieve customer demand precisely and enhance customer relations and organizational performance.
Nevertheless, in the future, there are opportunities for content recommendation engines to expand beyond AI/ML. This includes big data analytics, predictive analytics, AR for content delivery, and quantum computing. These evolving technologies will account for delivering a highly customized approach to the client.
Businesses must keep track of these technologies and improve their content recommendation approaches. This helps them to provide meaningful and relevant customer experiences and keep the organization ahead of the competition.